In our journey to build a robust monitoring system for our mysql instances on Azure, we encountered a significant challenge: Azure API rate limits. This blog post details how we leveraged Grafana Alloy with Azure Exporter to architect a more efficient monitoring solution.
How We Solved API Rate Limit Challenges with Grafana Alloy
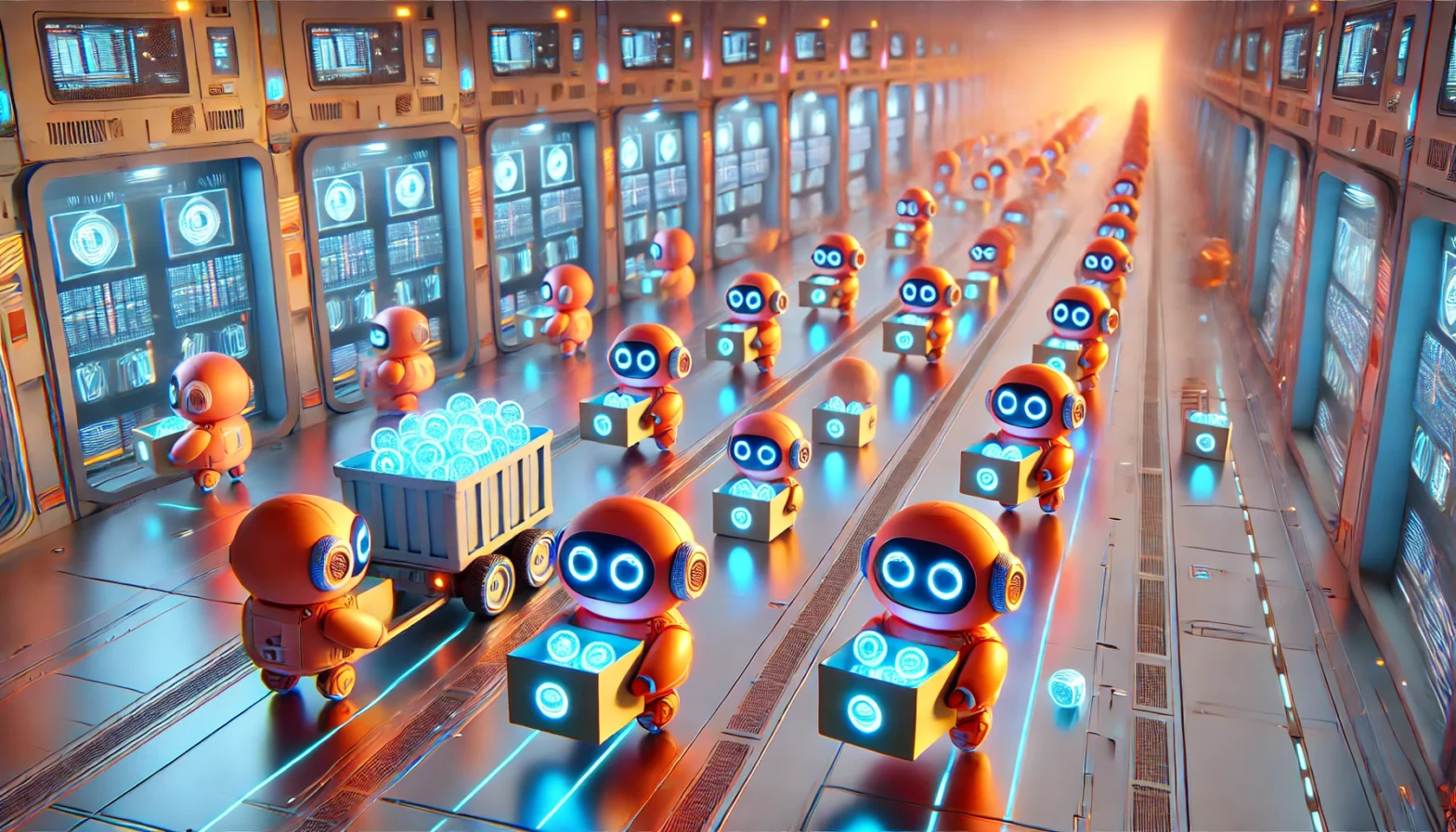